How AI for Sales Forecasting Can Revolutionize Your Business
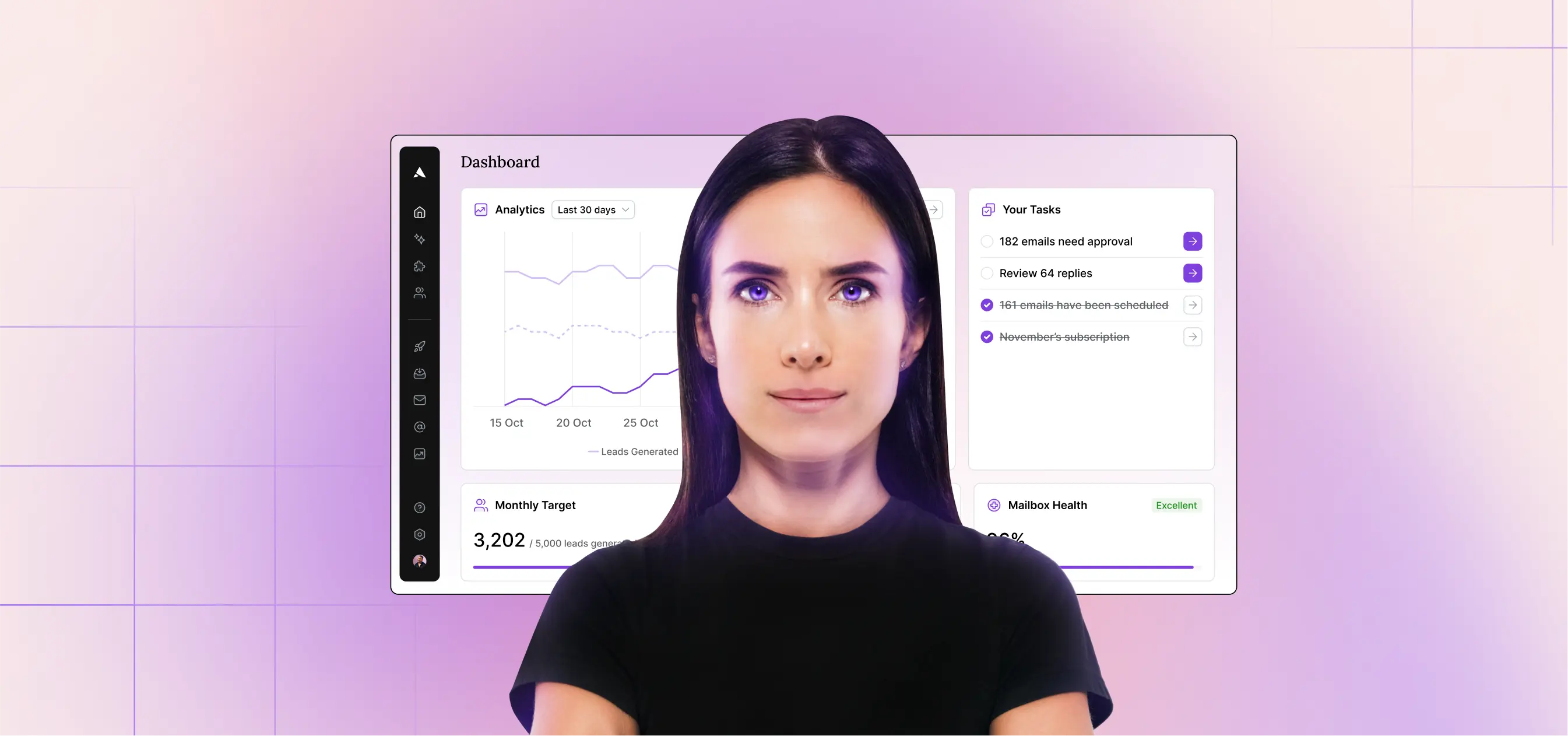
If you couldn’t trust the weather forecast to tell you whether it would be stormy or sunny, would you plan a grill party outdoors?Â
Not unless you like eating your burgers while soaking wet.Â
Sales predictions made the old-fashioned way can be just as unpredictable as weather reports. But it’s not a grill party that’s at stake. It’s everything from people’s jobs to your bottom line.
Luckily, there’s a better way: artificial intelligence.Â
AI forecasting tools analyze massive amounts of data, accurately predict future sales, and identify easy-to-miss trends.Â
Why Accurate Sales Forecasting Matters (With Practical Examples)
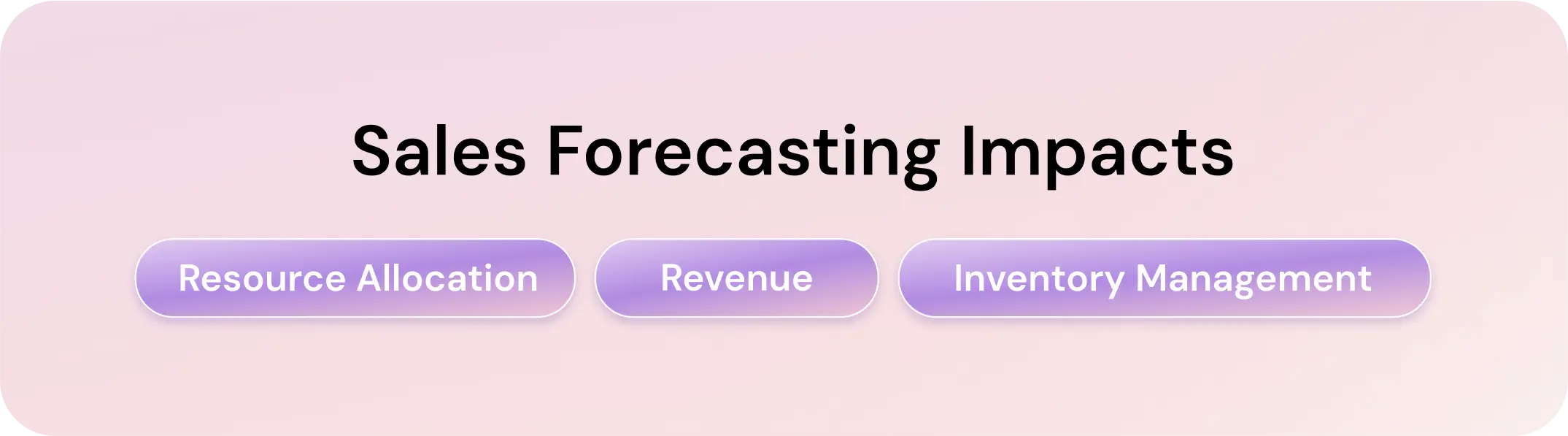
When sales forecasts are wrong, you end up spending money you don’t have or missing opportunities that could have driven growth.Â
This can lead to budget cuts or even layoffs if the forecast is too high. And overworked teams or lost market share if the forecast is too low.
Let’s look at the three main areas where sales forecasting can have a big impact.Â
Impact on Revenue
Forecasting accuracy helps you know how much money your company will make so you can plan properly.Â
Let’s say you forecast $500,000 in sales next year. But your team only brings in $350,000. You may have already dedicated that projected amount to hiring new employees, marketing, or expanding operations.Â
Only now, you’re short $150,000. Which means cuts need to be made somewhere to make up for it. On the flip side, if you only prepare for $350,000 but end up making $500,000, you’ve missed the chance to sell more, hire more, and grow faster. Which will hurt profitability.Â
Impact on Inventory ManagementÂ
For companies that sell physical products, forecasting helps you determine how much inventory to keep.Â
If the forecast is too high, you end up with extra stock sitting in storage, tying up cash and wasting resources that could’ve been better spent elsewhere.
If it’s too low, you won’t have enough products to meet demand and will lose out on sales.
Impact on Resource Allocation
If you’re unsure how much revenue is coming in, you can’t dedicate the proper resources to maintaining and growing the business.Â
If you expect a big sales increase, you might hire more staff or boost marketing. But if those sales don’t materialize, you're left overstaffed and overspent. Paychecks are at risk. And nobody wants a missed paycheck.
On the other hand, if you forecast too low and don’t hire or allocate enough staff, you may struggle to serve customers when demand picks up. This leads to longer wait times, missed orders, and frustrated customers—hurting your company’s reputation.
The Limitations of Traditional Sales Forecasting Methods
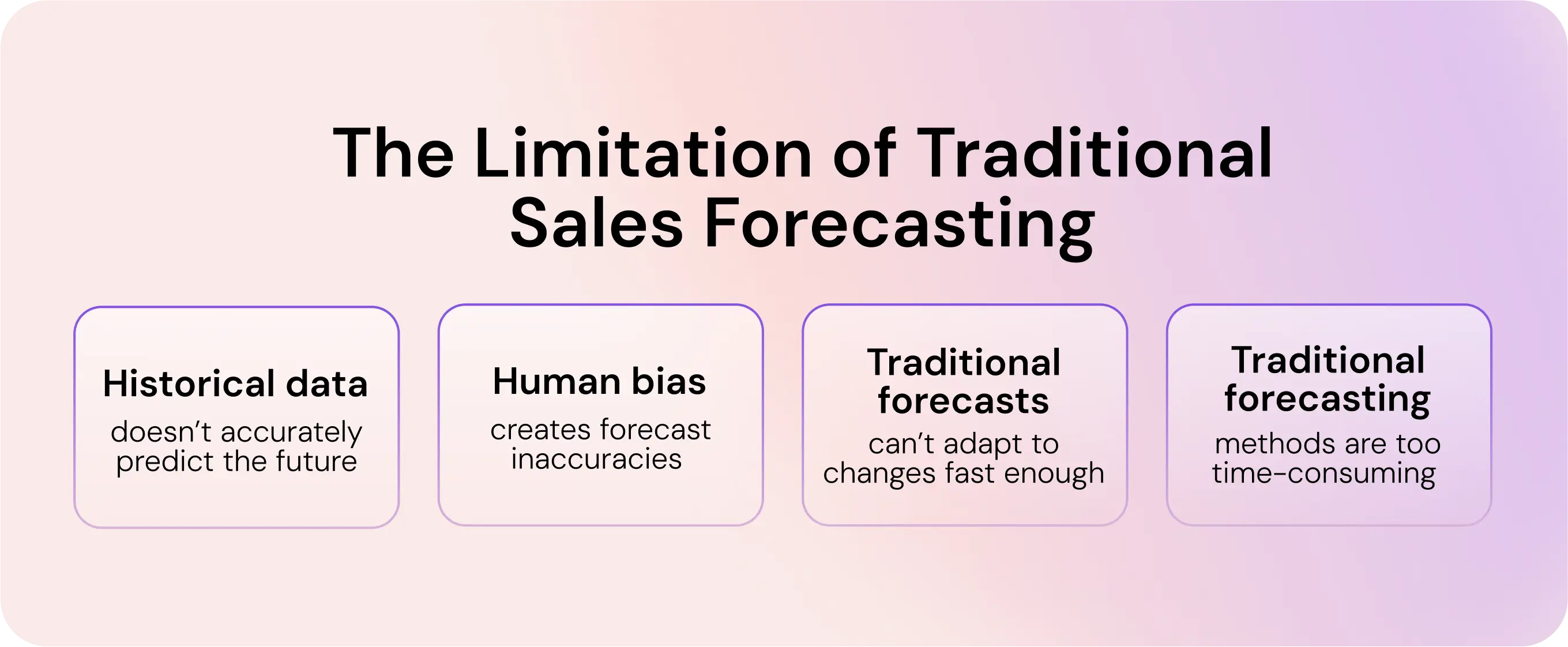
Traditional forecasting methods have four main limitations:Â
Historical data doesn’t accurately predict the futureÂ
Human bias creates forecast inaccuraciesÂ
Traditional forecasts can’t adapt to changes fast enough
Traditional forecasting methods are too time-consumingÂ
Let’s explore these in more depth.Â
Challenges With Historical Data AnalysisÂ
Traditional sales forecasting relies heavily on historical data to predict future performance.Â
But what worked in the past doesn’t always apply to the future. Markets change, customer behavior shifts, and relying too much on old data can lead to inaccurate projections.
For example, you might’ve based your 2022 forecasts on strong 2021 numbers. But since then, economic instability, inflation, and geopolitical tensions have disrupted the market.
Historical data alone can't account for sudden shifts, which leave you exposed when external factors change.
Human Bias in Sales ProjectionsÂ
Unfortunately, biases are a natural part of decision-making. And when sales team members make predictions based on personal feelings or past experiences, rather than objective data, you end up with inaccurate forecasts and decisions that don’t match reality.
For example, if a sales rep had a great quarter, they might feel confident (or want to impress management) and predict high numbers going forward. Even if the data doesn’t fully support that.Â
On the other hand, a bad sales period might cause them to be too cautious, expecting sales to stay low when there’s actually room for growth.
Limited Flexibility and AdaptabilityÂ
Traditional forecasting methods rely on fixed models that don’t easily adjust when things change. These models often assume that market conditions will stay the same. Which, let’s be honest, rarely happens.
Let’s say your company uses a spreadsheet-based forecasting model that relies on last year’s data to predict this year’s sales. If a sudden economic downturn hits, or a key supplier has a delay, the model doesn’t automatically adjust.
Instead, you need to manually update the forecast to factor in new conditions. And by the time you do that, the forecast might already be outdated.Â
This is one area where AI for sales forecasting trumps traditional models. Artisan’s AI sales agent Ava, for example, updates your forecasts in real time and adjusts your campaigns accordingly.
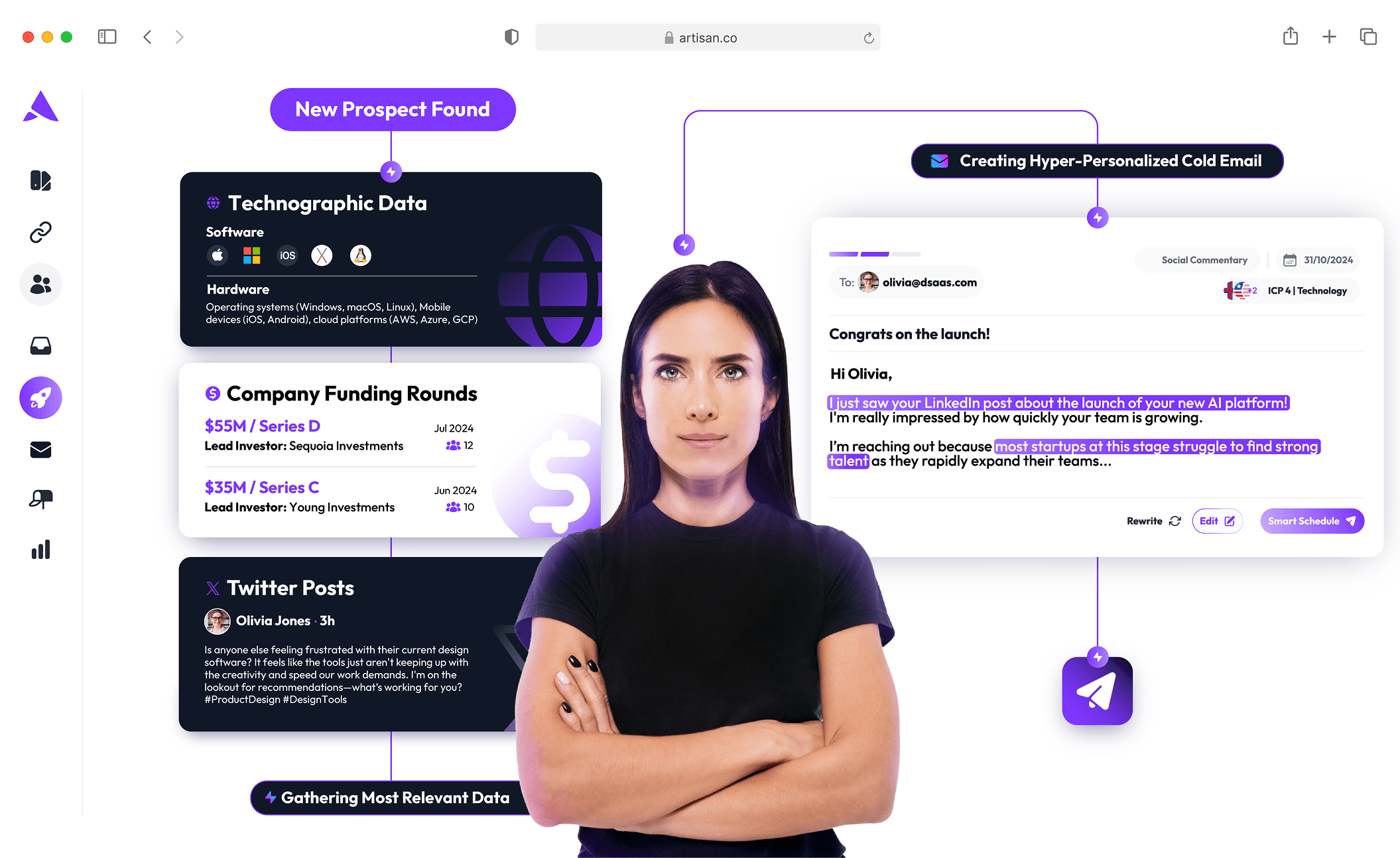
Want to see how Artisan's AI forecasting can help you reach more leads? Get in touch to schedule a demo.
Time-Consuming and Resource-Intensive ProcessesÂ
Traditional forecasting methods rely on people manually collecting and analyzing data. Salespeople spend hours gathering data from different sources and cleaning it up.Â
But they have other responsibilities too. Which slows down the process and diverts time away from more strategic tasks, like focusing on customer engagement or refining sales strategies.Â
Even when analysts are involved, they still need to find time to update forecasts as new data comes in, making the whole process slow and difficult to keep up to date. This delay slows decision-making, leaving companies far less agile.
How AI Is Transforming Sales Forecasting
According to Salesforce, four out of five sales teams were either experimenting with or had fully implemented AI in 2024.Â
And the results speak for themselves—83% of teams using AI saw revenue growth in 2024. Compared to just 66% of those who relied on traditional methods.
How did that happen?Â
AI-powered tools quickly analyze vast datasets and identify patterns humans often overlook. This gives AI-enabled sales teams higher-quality customer data and more accurate sales forecasts.Â
Here are the main ways that AI is transforming sales forecasting:
Basing predictions on a more diverse dataset: AI-powered sales tools factor in historical data, market trends, customer behavior, competitor information, and even macroeconomic factors like inflation in their predictions. Their knowledge isn’t limited by what’s available in your spreadsheets or CRM.
Analyzing data in real time for agile decision-making: AI tools continuously update forecasts as new information comes in, instead of relying on static, outdated data. So you can make the best decisions based on the latest insights.
Reducing bias and enhancing objectivity: AI algorithms make predictions based on the data they’re fed, not on gut feelings, personal experiences, or expectations. So their forecasts are more objective and data-driven.Â
Streamlining processes for increased efficiency: AI automates tasks like data collection and analysis, reducing manual labor. This frees up sales teams to focus on strategic activities, like improving customer satisfaction, especially in complex B2B sales.
Implementing AI for Sales Forecasting: A Step-by-Step Guide
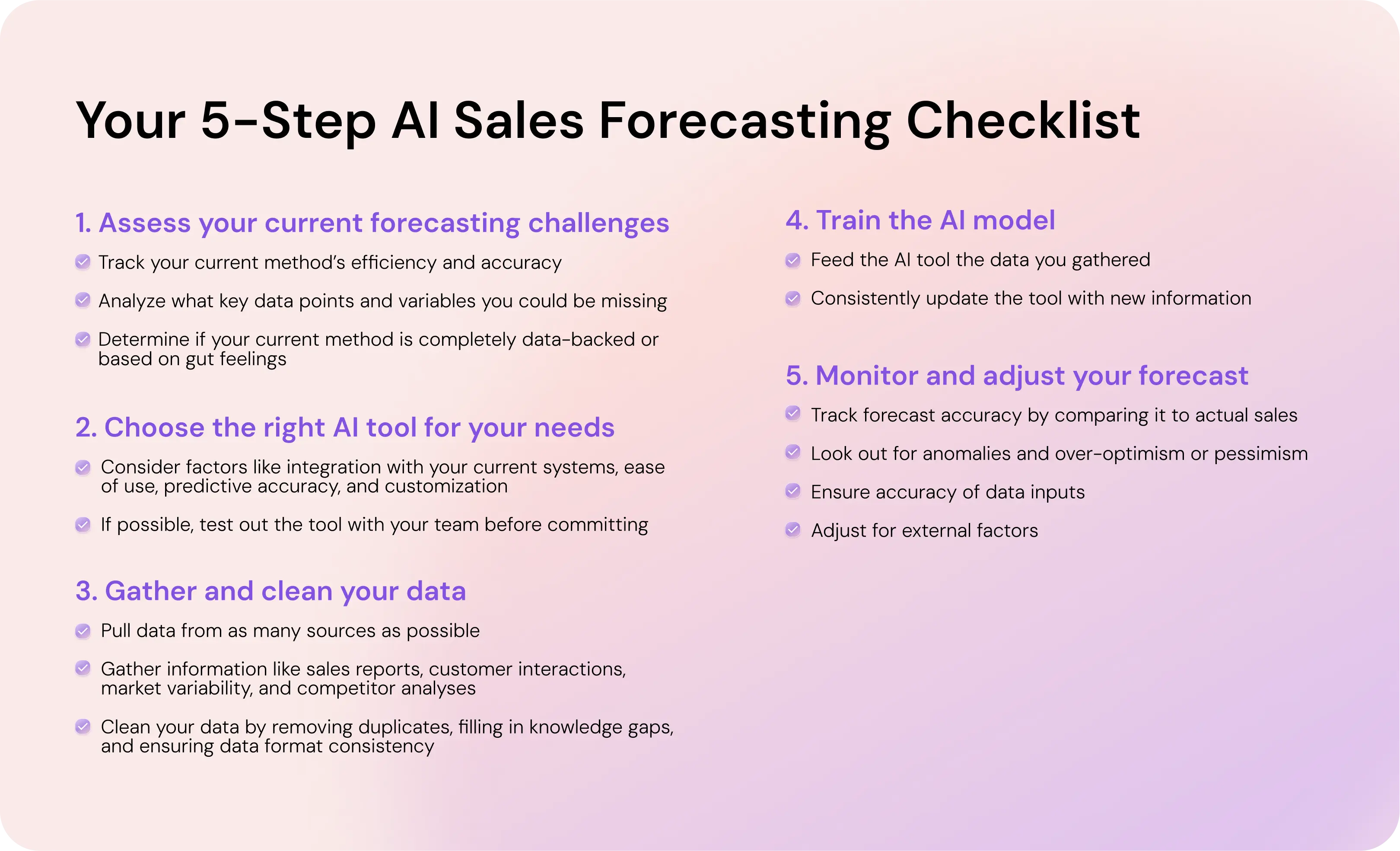
Considering implementing AI as part of your sales enablement strategy?Â
Fortunately, we’ve got a tested four-step strategy for integrating AI forecasting into your workflows quickly and cost-effectively.Â
Step 1: Assess Your Current Forecasting ChallengesÂ
Taking the time to assess your current forecasting methods helps you understand where you’re losing efficiency and accuracy.
To pinpoint your current forecasting challenges, ask yourself:
Are our forecasts consistently inaccurate?Â
Are we missing key market trends or customer behaviors?Â
Is our data spread across multiple platforms, making it harder to analyze?
Are we spending too much time manually compiling reports?
Are we relying on guesswork to make critical business decisions and set sales KPIs?
Answering these questions sets the foundation for AI to automate your processes and deliver more accurate, data-driven predictions—ultimately saving you time and improving your bottom line.
Step 2: Choose the Right AI Tools for Your NeedsÂ
Not all AI tools are created equal. In fact, there is significant variation between providers.Â
Here’s what to consider when picking AI forecasting tools:Â
Integrations: Ensure the tool easily integrates with your existing systems (like your CRM, sales analytics and reporting platform, and lead databases) either through native integrations or a custom API.
Ease of use: Even the most advanced AI won’t add value if it’s too complex for your team to adopt. If possible, request a personalized demo or free trial and try out the tool with your team before committing.Â
Predictive accuracy: Review case studies, customer testimonials, and industry reviews that highlight a tool’s forecasting accuracy. If you can, test the platform with your own data and compare its predictions against past sales forecasts.
Customization: Ensure the tool can be tailored to your specific needs. For example, it should let you focus on the sales metrics that matter most to your business. Or adjust forecasting models based on your sales cycle length, market conditions, or specific product lines.Â
Scalability: Choose a solution that grows with you so you don’t have to switch systems down the line.
Artisan’s AI sales rep Ava, for example, collects data on every stage of the sales funnel to create personalized email sequences at scale.
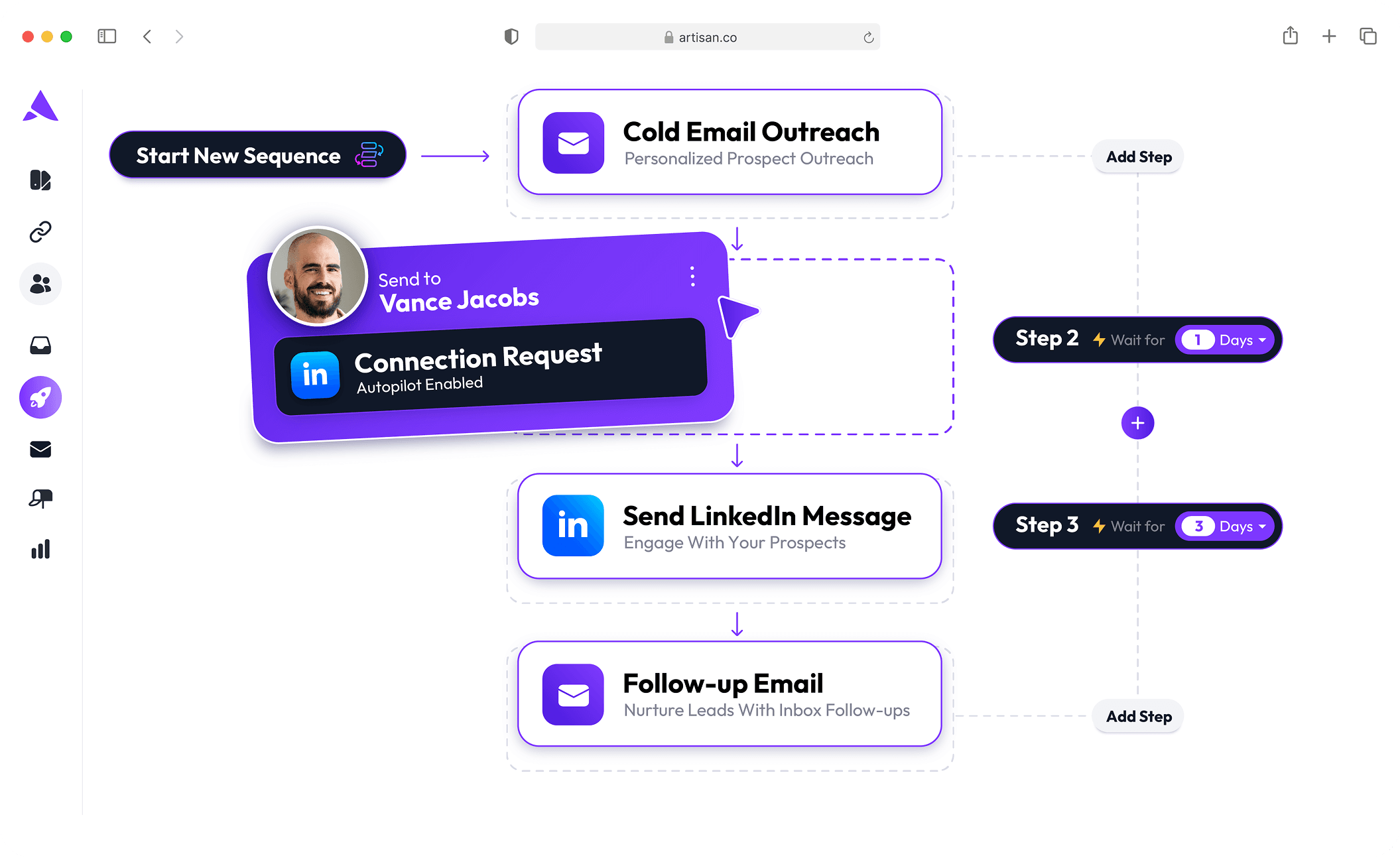
Want to see how AI forecasts can help you hit revenue targets and save resources? Get in touch to schedule an Artisan demo. Â
Step 3: Gather and Clean Your DataÂ
You need to train generative AI models on as much information as possible if they are to learn patterns and generate accurate predictions tailored to your business.
Gather AI training data from the following sources:
Sales reports
Customer interactions (including information on their behavior and preferences)
Data on market trends and seasonality
Insights on competitorsÂ
Pull data from everywhere: your CRM, marketing platforms, even your finance systems. The more connected and complete your data, the better your AI forecasts will be.
Next, clean and organize your data. This means removing duplicates, filling in gaps to ensure a complete dataset, and ensuring everything is consistent. For example, dates should all follow the same format, currency symbols should be uniform, and all fields should be standardized.Â
Step 4: Train the AI Model With Your Data
After gathering your data, you’ll need to train the AI model.Â
This involves “feeding” it the data you gathered and cleaned in the previous step. Once it learns about your business (like your past sales performance) and your market, it can start predicting future sales.Â
Remember that training AI is an ongoing process. The more data AI forecasting tools take in from both past and present conditions, the more accurate and reliable their predictions become.
Step 5: Monitor and Adjust Your Forecast
AI sales forecasting tools make powerful predictions, but a “set it and forget it” approach won’t work.Â
You need to monitor how well the forecasts align with actual results and make adjustments when necessary.Â
Here’s how to adjust your forecasts:Â
Track forecast accuracy: Compare forecasted sales with actual sales each month or quarter. Significant gaps mean something’s off.
Monitor data inputs: Ensure the data feeding the AI is still relevant. Market conditions change, and so should the data you provide.
Spot anomalies: If the forecast suddenly swings dramatically without a clear reason, dig into what’s causing that. It could mean the model needs tweaking.
Watch for over-optimism and pessimism: If your AI consistently predicts overly high or low sales, it might need recalibration. This could happen if new data isn’t integrated well.
Adjust for external factors: Make sure external factors like economic shifts are being accounted for in the forecasts. If they're not, the predictions will miss the mark.
Embrace AI for a Competitive Edge
Traditional sales forecasting methods hold sales teams back. Reps spend hours setting up a forecast only for it to become obsolete when market conditions shift.Â
That’s why so many sales leaders are turning to AI. AI gives accurate, real-time insights and automatically adjusts sales data to new market conditions. This means reps can focus on strategic, human tasks, confident that their forecasts are always up-to-date and relevant.
Want to see how Ava, Artisan’s AI BDR, can help you forecast faster and more accurately? Get in touch to schedule a demo. book a demo today.